Can AI Help Address Specialty Crop Growers’ Critical Needs?
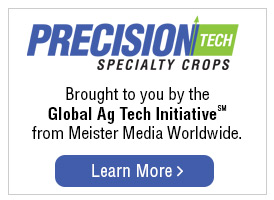
With the recent release of ChatGPT creating a buzz about the potential power and risks of artificial intelligence (AI), many readers may be wondering how AI might make a meaningful contribution to agriculture. Or is AI just another example of emerging technologies that never quite provide cost-effective benefits?
You may recall the early excitement around AI’s potential to detect objects in images from satellites, drones, or mobile phones. Despite promising claims of it reducing costs and time by pinpointing impacts of pests, diseases, or irrigation issues, few cost-effective applications emerged as widespread, standard practices.
That’s changing. Today, image analysis with AI is, in fact, useful for specialty crop agriculture. Developers are learning how to take the technologies from the lab to the field. Think of computer vision that detects weeds for robotic weeders and counts plants for robotic thinning.
These applications make economic sense in the face of increasing labor costs and shortages. As growers face additional pressures from increasingly stringent regulations, rising input costs, and buyer demands for products grown in accordance with guidelines on sustainability, additional applications of AI are bound to emerge, provided they help growers save time or money, or help them meet regulatory or buyer demands.
Our team worked with another application of this technology. We used aerial imagery and AI to demonstrate the potential value of measuring postharvest loss in sweet potato fields for a project wrapping up in North Carolina.
A drone collected high-resolution images immediately following harvest, and AI algorithms analyzed the images to detect sweet potatoes remaining partially buried or on the surface.
The results were promising. AI detected most of the unharvested sweet potatoes, providing a measure of the field’s overall production efficiency — how much of the planted crop was indeed harvested and sold.
How It Works
Developing the algorithm took time. Images of all types of conditions in previously harvested fields “trained” the AI to “see” and count only sweet potatoes and exclude rocks, vines, clumps of soil, footprints, and bucket prints. All of which can look surprisingly like a sweet potato.
We used two filters to differentiate the sweet potatoes:
RGB (red-green-blue) and NIR (near-infrared). Each filter has strengths and weaknesses, so we needed both.
Soil moisture influences the results, because these filters notice the moisture in the sweet potato. With moist soil, sweet potatoes are harder to notice.
For the next training step, we labeled the filtered images with bounding boxes around each sweet potato, then had software using segmentation identify the sweet potato within that box. Only then can we estimate the size and economic value of the remaining crop.
Once the algorithm is trained, analyzing an image takes less than a minute.
There are many reasons why we grow crops to maturity but don’t sell them — size, quality, pest damage, and market conditions that may not justify harvest costs. But it’s still worth your time to track what’s left behind. The old adage of “what gets measured gets managed” can guide future crop planning and mid-season decision making.
You can also use this approach after the sweet potatoes are turned over prior to harvest. That allows you to compare how losses impact the final yield, providing insights about overall production efficiency.
In-field sensors can provide additional input. By now, anyone who enters a field with their mobile device has the potential to collect details on production in the form of geo-positioned photos, notes, and connections to additional sensors.
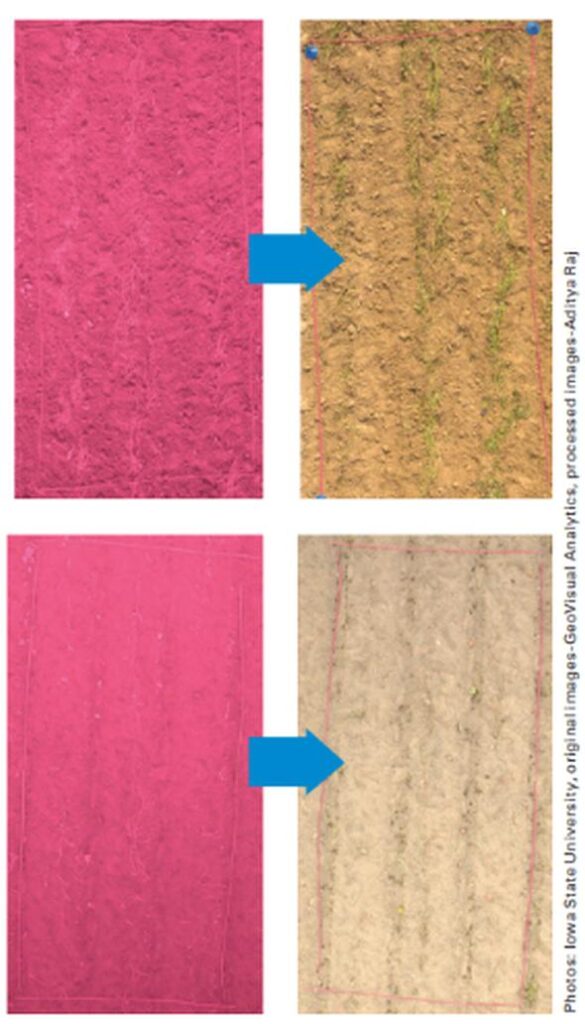
The AI algorithm needed two filters to detect the sweet potatoes. Notice what you can see and not see between these two different field sections.
Photos: Iowa State University, original images-GeoVisual Analytics, processed images-Aditya Raj
As the amounts of production-related data increase, AI algorithms will no doubt help make sense of them — to better analyze the causes of and remedies for typical production loss factors.
With the rapid pace of AI advancements, the extent to which they will help address growers’ pressing needs will depend on how effectively they can be applied in the complex, real-world environment of a production farm. Those technologies that do emerge as standard practices no doubt will have been developed in close collaboration with the production teams that use them.
Projects like the one in North Carolina help the industry collect and analyze information on the factors impacting production and have the potential to make the supply chain more efficient, less susceptible to losses in the field, and ultimately more resilient and stable for the future.